Trustworthy human-robot interaction
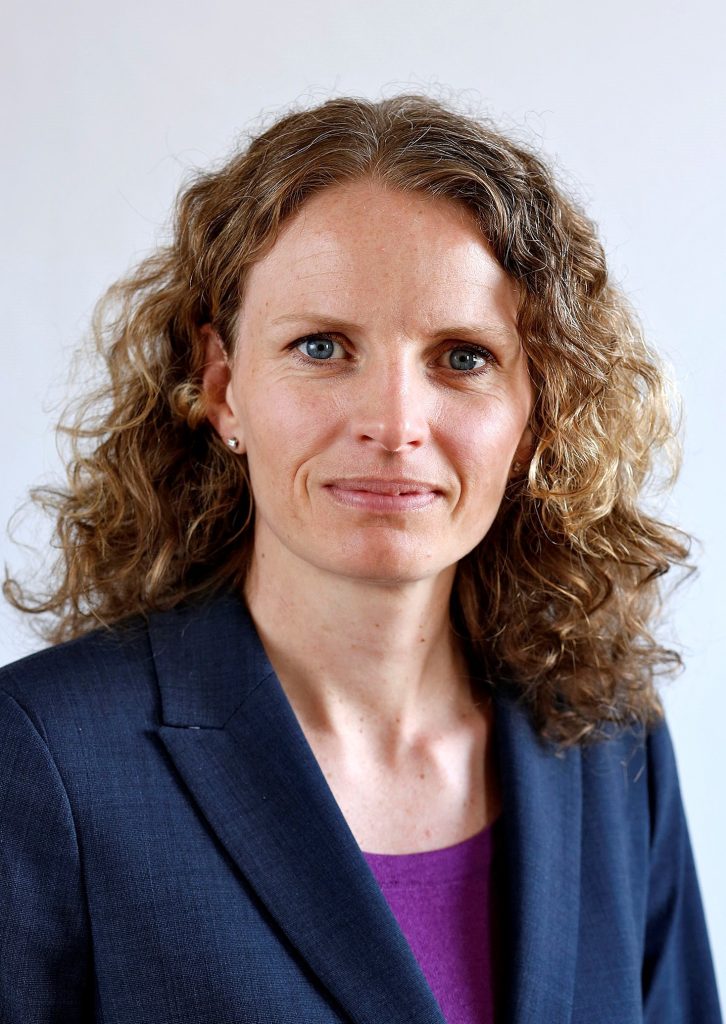
Head of School of Informatics at the University of Edinburgh, Scotland, United Kingdom
Abstract
Trust is a multifaceted, complex phenomenon that is not well understood when it occurs between humans, let alone between humans and robots. Robots that portray social cues, including voice, gestures and facial expressions, are key tools in researching human-robot trust, specifically how trust is established, lost and regained. In this talk, I will discuss various aspects of trust for HRI including language, social cues, embodiment, transparency, mental models and theory of mind.
Biography
Helen Hastie is the Head of the School of Informatics of the University of Edinburgh and a RAEng/Leverhulme Trust Senior Research Fellow. She specialises in Human-Robot Interaction and Multimodal interfaces. Hastie has undertaken projects such as AI personal assistants for remote robots, autonomous systems and spoken dialogue systems for sectors in defence and energy. She is a Fellow of the Royal Society of Edinburgh.
Human-Robot interaction with Large Language Models
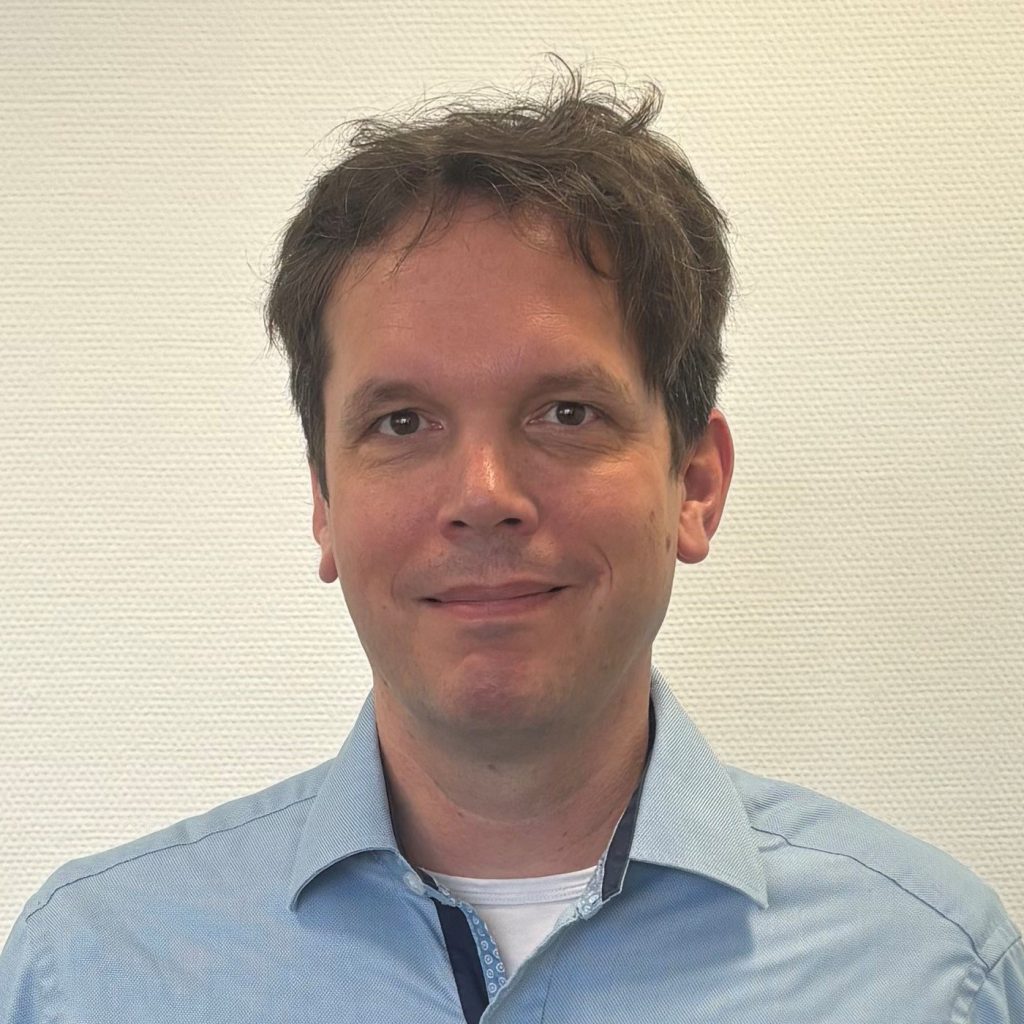
Michael Gienger
Chief Scientist at Honda Research Institute, Offenbach, Germany
Abstract
The recent breakthroughs in Generative AI offer fantastic opportunities to research novel concepts for intelligent embodied agents. In this keynote, I will introduce our findings in designing interactive robot agents that learn from humans, and that can exploit their acquired knowledge in different situations. We designed a Virtual Playground, in which we conducted user studies to understand the efficiency of robot curiosity as well as of multi-modal cues with respect to an explainable interaction. To close the gap towards behavior generation, I will introduce recent research in exploiting Large Language Models (LLMs) for robot task and motion planning. We combined reasoning, planning, and motion generation, and introduced a novel concept for correcting errors during planning and execution. I’ll show several results both in simulations and real-world tasks for tasks like block arrangement, cocktail, and pizza preparation. I will then discuss our recent concept of “Attentive Support”, in which we made the step from LLM-based autonomous problem-solving capabilities to human-robot group constellations and conclude with my view of interesting future research questions.
Biography
Michael Gienger received the diploma degree in Mechanical Engineering from the Technical University of Munich, Germany, in 1998. Until 2003, he was research assistant at the Institute of Applied Mechanics of the TUM and received his PhD degree with a dissertation in the field of robotics. After this, he joined the Honda Research Institute Europe in Germany, where he currently holds a position as a Chief Scientist and Competence Group Leader in the field of robotics. His research interests include mechatronics, robotics, whole-body control, imitation learning, and human-robot interaction.